Understanding Annotation in Machine Learning: A Key to Business Innovation
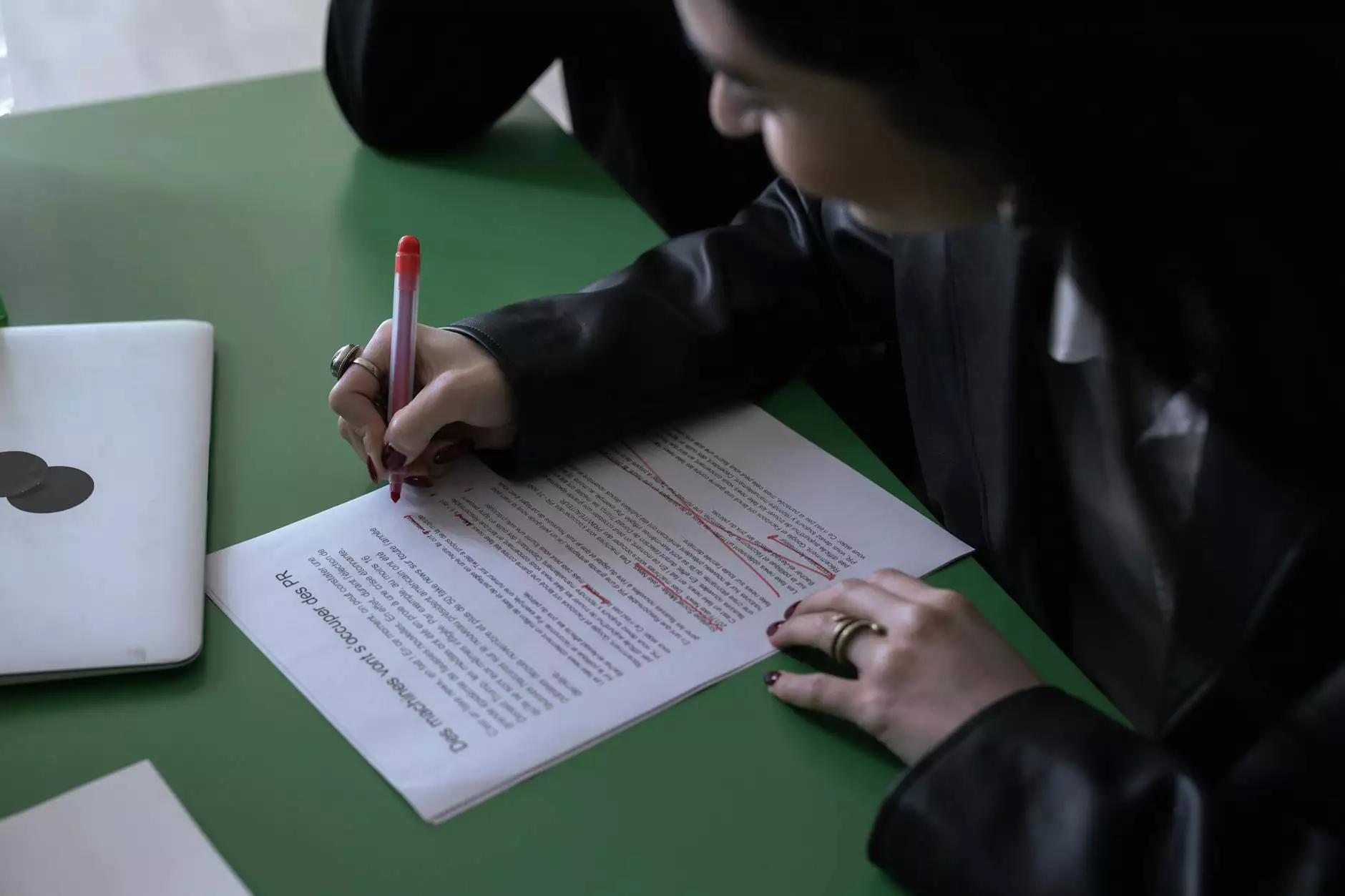
In today's competitive landscape, businesses are constantly seeking ways to leverage the latest technological advancements to gain an edge. One such advancement is annotation in machine learning, a crucial process that enables organizations to harness the power of data for improved insights and decision-making. In this comprehensive guide, we will explore what annotation in machine learning entails, its significance for various business sectors, particularly focusing on home services and keys & locksmiths, and how it can drive innovation and success.
The Fundamentals of Annotation in Machine Learning
At its core, annotation in machine learning refers to the process of labeling data to train algorithms so they can learn and make predictions. This process is essential for machine learning models, particularly supervised learning, where models need labeled input data to draw inferences from. The accuracy and effectiveness of these models hinge significantly on the quality and precision of the annotations provided.
Types of Data That Require Annotation
To fully understand how annotation plays a pivotal role in machine learning, it’s important to look at the types of data that necessitate this process:
- Image Data: In applications like facial recognition, autonomous vehicles, and even locksmith services needing identification verification, images are annotated with labels outlining specific features.
- Text Data: For businesses engaging in customer service or content management, text annotation includes categorizing reviews, extracting entities, or understanding sentiments expressed in customer feedback.
- Audio Data: This involves annotating recordings for speech recognition systems, which is beneficial for enhancing customer service interfaces.
The Importance of High-Quality Annotations
Quality annotations are vital for the success of machine learning models. Poorly labeled data can lead to inaccurate predictions, which in turn can jeopardize business operations. Hence, investing in high-quality data annotation is crucial.
Business Benefits of Annotation in Machine Learning
The integration of machine learning through effective annotation brings forth numerous advantages for businesses, particularly in the home services and locksmith sectors. Here are some key benefits:
1. Enhanced Customer Experiences
With accurate data-driven insights derived from annotated data, businesses can tailor their services to meet customer needs effectively. For instance, in the locksmith industry, employing machine learning analytics can help understand peak demand times and customer preferences, enabling businesses to offer personalized services.
2. Improved Operational Efficiency
Machine learning models that utilize annotated data can optimize operations significantly. For instance, predictive maintenance can be established in home services by analyzing historical data, which can preemptively address equipment failures before they occur, saving time and resources.
3. Building Innovative Products
Annotations facilitate the development of smart products and services. For example, locksmiths can integrate AI-based solutions that utilize annotated datasets for smart lock technologies, offering customers enhanced security features coupled with convenience.
4. Competitive Edge
Organizations that harness the power of machine learning through effective annotation stand out in the crowded marketplace. By developing unique solutions and superior customer service capabilities, companies can solidify their reputation and grow their market share.
Implementing Annotation in Machine Learning
Integrating annotation within a machine learning framework requires a strategic approach. Below are crucial steps to consider:
1. Define the Purpose
Businesses need to begin by clearly defining what they aim to achieve with their machine learning models. Identifying specific use cases is essential—be it improving customer service, enhancing security, or automating processes.
2. Choose the Right Tool for Annotation
With multiple annotation tools available, businesses should choose one that aligns with their needs. Factors to consider include the type of data, expected output, and user-friendliness of the tool.
3. Establish Guidelines for Annotation
Creating clear guidelines can help maintain consistency and accuracy in the annotations. This includes defining what labels to use and how to categorize them effectively.
4. Quality Assurance
Implementing a quality assurance process is crucial. Regularly reviewing annotated data will ensure high quality, which is paramount for successful model training.
Challenges of Annotation in Machine Learning
While the advantages of annotation in machine learning are vast, there are also various challenges businesses may encounter:
1. Time-Consuming Process
The process of annotating data can be labor-intensive and time-consuming. Companies often struggle to manage large datasets efficiently, which can delay project timelines.
2. Requirement for Expertise
Quality annotations require skilled personnel who understand the domain well. Businesses may face difficulties in hiring or training annotators with the necessary knowledge.
3. Ensuring Consistency
Maintaining consistency across annotations, particularly with larger teams, can pose challenges. Clear guidelines and regular training sessions are essential to minimize discrepancies.
Future Trends in Annotation for Machine Learning
As technology continues to evolve, the landscape of annotation in machine learning will also change. Here are some future trends to watch:
1. Automation of Annotation Processes
With the advances in AI and technology, automated systems for annotation are likely to become more prevalent. This would significantly reduce time and costs associated with manual annotation.
2. Crowdsourced Annotation
Leveraging crowdsourcing techniques for data annotation can provide businesses access to a large pool of annotators, improving efficiency and scalability.
3. Increasing Role of AI in Annotation
AI will play a bigger role in assisting with data annotation tasks. For instance, active learning techniques can improve the quality of annotations by allowing models to suggest labels for unannotated examples.
Conclusion: Embracing Annotation in Machine Learning for Business Growth
In conclusion, annotation in machine learning is a pivotal concept that can transform how businesses operate, particularly in the home services and locksmith sectors. By understanding the process, benefits, challenges, and future trends associated with data annotation, organizations can harness its potential to foster innovation, improve efficiency, and ultimately, achieve sustainable growth. As the marketplace becomes increasingly reliant on advanced technologies, those willing to embrace annotation and machine learning will not only survive but thrive.
For businesses like KeyMakr, investing in high-quality annotation processes can be the key to unlocking new opportunities and enhancing customer satisfaction. It’s time to take step forward, embrace the future, and lead the way in your industry.