Maximizing Efficiency with Video Labeling Tool Machine Learning
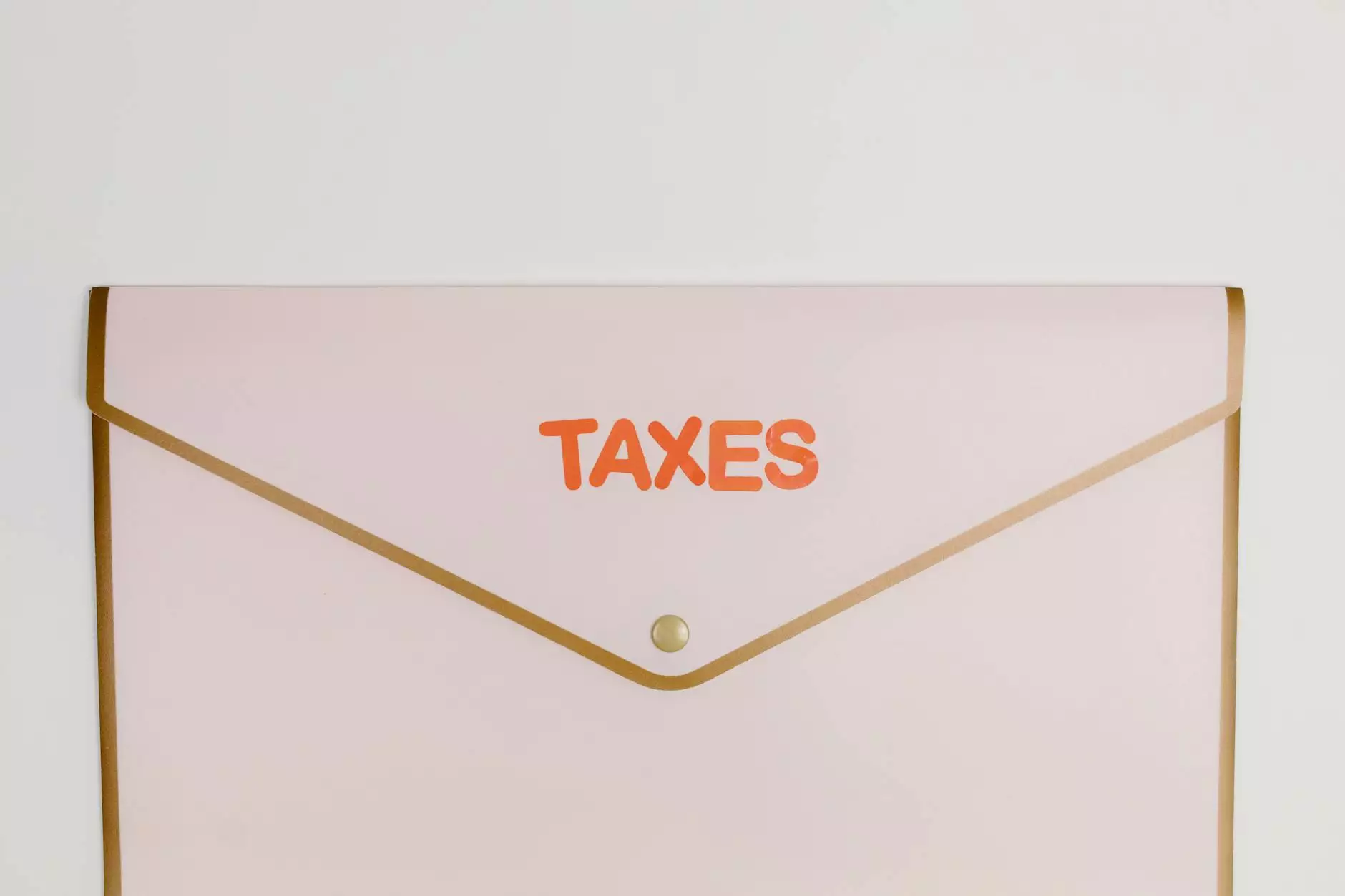
In the fast-paced world of technology, businesses are continuously searching for ways to improve operational efficiency. One sector that has seen remarkable advancements is data annotation, particularly in the realm of video processing. The emergence of a video labeling tool machine learning has transformed the way companies handle large volumes of video data, enhancing both accuracy and productivity.
Understanding Video Labeling Tools
Video labeling tools are software solutions designed to assist in the annotation of video content. Annotation involves adding metadata to video clips, making it easier for machine learning algorithms to understand and interpret this data. The integration of machine learning in these tools significantly enhances their capabilities, automating cumbersome processes while maintaining high levels of accuracy.
Why is Video Annotation Important?
The importance of video annotation extends beyond mere labeling; it plays a critical role in various applications, including:
- Object recognition: By training models to identify and classify objects within videos, businesses can develop advanced AI systems for numerous applications.
- Autonomous vehicles: Accurate video labeling is essential for building self-driving car technology, ensuring safety and efficiency on the roads.
- Surveillance systems: Enhanced video analytics can empower security systems to monitor and assess environments effectively.
- Healthcare: Video analysis can aid in medical diagnostics, such as analyzing surgical procedures or patient movements.
- Sports analytics: Coaches and analysts can break down plays and performance through detailed video data assessment.
Features of Advanced Video Labeling Tools
Modern video labeling tool machine learning solutions come equipped with a variety of powerful features designed to streamline the video annotation process:
1. Automation and Efficiency
Automation is a standout feature in machine learning-driven video labeling tools. These tools can automatically detect and label objects within a video, significantly reducing the time and effort required for manual labeling. This not only speeds up the annotation process but also allows human annotators to focus on more complex tasks that require critical thinking.
2. High Accuracy and Consistency
One of the challenges faced in traditional video labeling is the risk of human error. A video labeling tool machine learning mitigates this risk by providing consistent and accurate annotations across large datasets. Algorithms can learn from previous annotations, improving their precision with each iteration and minimizing discrepancies that could lead to inaccurate model training.
3. Real-time Processing
With the capability for real-time video processing, businesses can receive immediate insights and updates. This feature is particularly valuable for industries such as security and surveillance, where timely decision-making is crucial to operations.
4. User-Friendly Interface
Despite their advanced capabilities, many video labeling tools are designed with user-friendliness in mind. Intuitive dashboards and graphical interfaces allow users to navigate the software easily, facilitating a seamless transition from traditional methods to machine learning-enhanced processes.
Implementing Video Labeling Tools in Your Business
To successfully adopt a video labeling tool machine learning, businesses should follow a structured implementation process. Here are essential steps to consider:
1. Identify Your Needs
Before selecting a video labeling tool, assess your specific requirements. Determine the volume of video data you handle, the types of annotations needed, and the desired outcomes of implementing machine learning.
2. Choose the Right Tool
Not all video labeling tools are created equal. Research and compare various solutions, considering functionality, scalability, user reviews, and price. KeyLabs.ai offers robust data annotation platforms suited for businesses of all sizes, ensuring comprehensive solutions tailored to your needs.
3. Train Your Team
Invest in training your team to maximize the benefits of your new tool. Familiarize them with the software's features, functionalities, and best practices for effective video labeling. Empowering your team will encourage efficiency and foster innovation.
4. Start Small and Scale Up
Begin with smaller projects to test the effectiveness of the video labeling tool. As your team gains confidence and efficiency, gradually scale up to more complex data sets and diverse video content.
Case Studies: Success Stories with Video Labeling Tools
Real-world applications speak volumes about the effectiveness of video labeling tool machine learning solutions. Here are some success stories from various sectors:
1. Automotive Industry
A leading automotive manufacturer implemented a video labeling tool to enhance its self-driving car technologies. By efficiently labeling thousands of hours of road footage, the company significantly accelerated the development of its autonomous system, leading to improved safety features and reduced testing costs.
2. Retail Sector
In the retail space, a major chain utilized video analytics coupled with labeling tools to assess customer behavior in-store. By understanding foot traffic patterns, the business optimized store layouts and inventory placement, resulting in increased sales and improved customer satisfaction.
3. Healthcare Innovations
A healthcare technology firm leveraged video labeling tools for analyzing surgical procedures. By annotating video footage, they developed machine learning models capable of assessing surgical techniques, ultimately enhancing training programs and improving patient outcomes.
Future Trends in Video Labeling Tool Machine Learning
The landscape of video labeling is continually evolving, driven by technological advancements and a growing demand for efficiency. Here are some future trends to watch:
- Augmented Reality (AR) Integration: Expect to see more AR features in video labeling tools, allowing users to interactively annotate video content in immersive environments.
- Continuous Learning: Future tools may integrate enhanced algorithms that continuously learn and adapt based on user feedback and new data inputs, further increasing accuracy over time.
- Greater Customization: Tools will offer more personalized experiences, allowing businesses to tailor their video labeling processes to meet specific industry needs.
- Collaborative Platforms: Enhanced collaboration features will allow teams to work together on annotation tasks in real-time, fostering innovation and creativity.
Conclusion
In today's data-driven world, the value of a robust video labeling tool machine learning cannot be overstated. These tools not only save valuable time and resources but also provide businesses with the competitive edge required in their respective industries. As you consider integrating such technology into your operations, remember that with the right approach, you can maximize the efficiency and effectiveness of your data annotation efforts.
For more information and to explore the best video labeling tools available, visit KeyLabs.ai. Equip your business with the cutting-edge technology it deserves and stay ahead in the ever-evolving landscape of data annotation.