Labeling Training Data: The Key to Quality AI Models
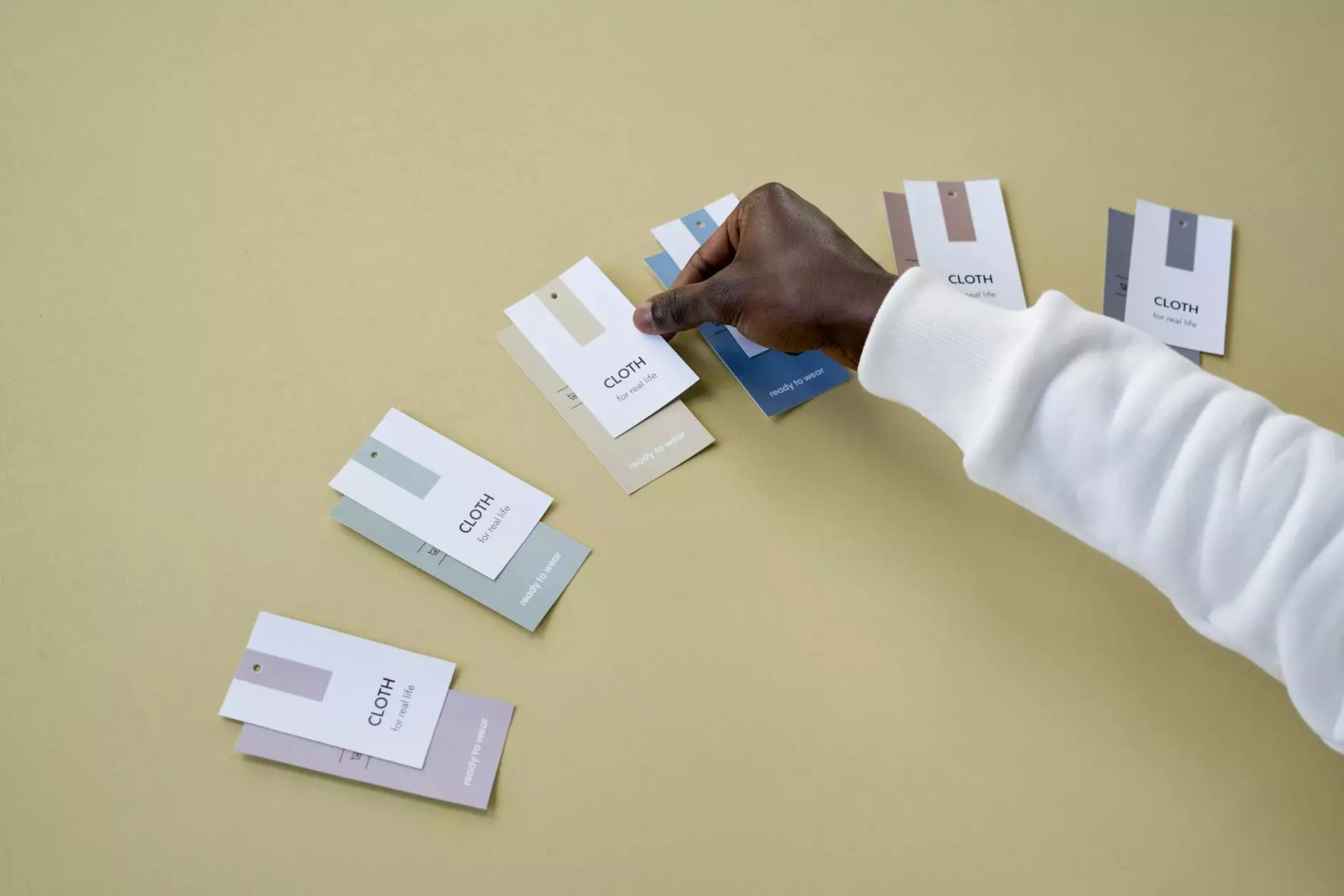
Labeling training data is a critical step in developing robust and reliable machine learning models. In the world of artificial intelligence (AI), the effectiveness of a model is largely determined by the quality of data it is trained on. Without proper data annotation, even the most advanced algorithms can fail to produce accurate results. In this article, we will explore the importance of labeling training data, best practices, and how the leading solutions from KeyLabs.ai can revolutionize your AI projects.
The Importance of Labeling Training Data
Labeling training data involves the process of categorizing and tagging data points to provide contextual information that machines can learn from. This is fundamental for various applications such as:
- Image Recognition: Identifying objects, faces, or scenes.
- Natural Language Processing (NLP): Understanding and generating human language.
- Speech Recognition: Converting spoken language into text data.
- Healthcare Data Analysis: Diagnosing diseases from medical images or patient records.
How Accurate Labeling Enhances Model Performance
When it comes to training AI models, accuracy is paramount. Properly labeled training data influences model performance in several ways:
- Improved Prediction Accuracy: Well-labeled data allows models to learn more effectively, reducing error rates.
- Increased Robustness: With diverse and accurately labeled data, models can generalize better to new, unseen data.
- Faster Training Times: Consistent labeling standards streamline the training process, allowing for quicker iterations.
- Enhanced Insights: Accurate labels provide clearer insights from the data, fostering better decision-making.
Best Practices for Labeling Training Data
To maximize the effectiveness of your data annotation efforts, consider the following best practices:
1. Define Clear Labeling Guidelines
Creating comprehensive labeling guidelines is essential. These should include:
- Specific Definitions: Clearly delineate what each label means.
- Examples: Provide plenty of examples to illustrate correct and incorrect labeling.
- Quality Metrics: Establish metrics to evaluate labeling consistency and accuracy.
2. Choose the Right Tools and Technologies
Selecting the appropriate data annotation tools can significantly impact the quality of your labeled data. Look for solutions that offer:
- User-Friendly Interface: Tools should be easy to use for annotators.
- Scalability: The ability to handle large datasets efficiently.
- Collaboration Features: Facilitate teamwork among annotators and data scientists.
- Machine Learning Assistance: Tools that use ML techniques to assist in the labeling process can enhance accuracy.
3. Implement a Review Process
A robust review process is vital to ensure data quality. This can include:
- Quality Control Checks: Regular audits of labeled data to identify errors.
- Feedback Mechanisms: Provide annotators with feedback to improve their performance.
- Multiple Annotators: Utilize multiple people to label the same data points to reduce bias.
4. Utilize Automation Where Possible
Advancements in AI and machine learning have led to the development of automated labeling solutions. These can:
- Save Time: Automating routine labeling tasks can free up resources for more complex tasks.
- Provide Consistency: Automated processes can ensure consistent application of labels.
- Support Hybrid Approaches: Combine automated and manual efforts to leverage strengths of both methods.
KeyLabs.ai: Your Partner in Data Annotation
When it comes to labeling training data, partnering with the right provider can make all the difference. KeyLabs.ai stands out with its innovative Data Annotation Platform designed to meet the needs of various industries:
Advantages of Using KeyLabs.ai
- Customizable Solutions: Tailor the annotation process to fit the specific requirements of your project.
- Expert Annotators: Access a team of skilled annotators who understand the nuances of your domain.
- Advanced Technologies: Incorporate state-of-the-art AI tools that enhance the speed and accuracy of data labeling.
- Comprehensive Support: Enjoy around-the-clock support to address any issues or questions during the process.
Industries Served
KeyLabs.ai's data annotation tools are versatile and cater to a wide range of industries, including:
- Healthcare: Medical image labeling, patient data classification.
- Automotive: Self-driving car data annotation.
- Retail: Image recognition for products, sentiment analysis for customer reviews.
- Finance: Fraud detection data labeling.
Future Trends in Labeling Training Data
As AI continues to evolve, the processes surrounding labeling training data are likely to undergo significant changes. Key trends include:
1. Increased Use of AI in Annotation
We can expect to see an uptick in AI-driven annotation tools that automate tedious aspects of the labeling process while maintaining accuracy.
2. Enhanced Collaboration Tools
With the growing emphasis on remote work, tools that facilitate real-time collaboration among teams will become increasingly important.
3. Emphasis on Ethical AI
As organizations strive for more ethical AI systems, data labeling will need to consider fairness and bias mitigation from the ground up.
4. Focus on Data Privacy
As data regulations tighten globally, ensuring compliance in the labeling process will be crucial for organizations in any industry.
Conclusion
In the competitive landscape of AI and machine learning, the significance of labeling training data cannot be overstated. Properly labeled data not only enhances model performance but also plays a crucial role in delivering insights that drive business strategies. With the right tools and practices in place, organizations can ensure high-quality training data that leads to successful AI initiatives. Discover how KeyLabs.ai can elevate your data annotation process and help you unlock the full potential of your AI projects.